Video vs. Audio Summarization Techniques and Challenges

Dictationer
•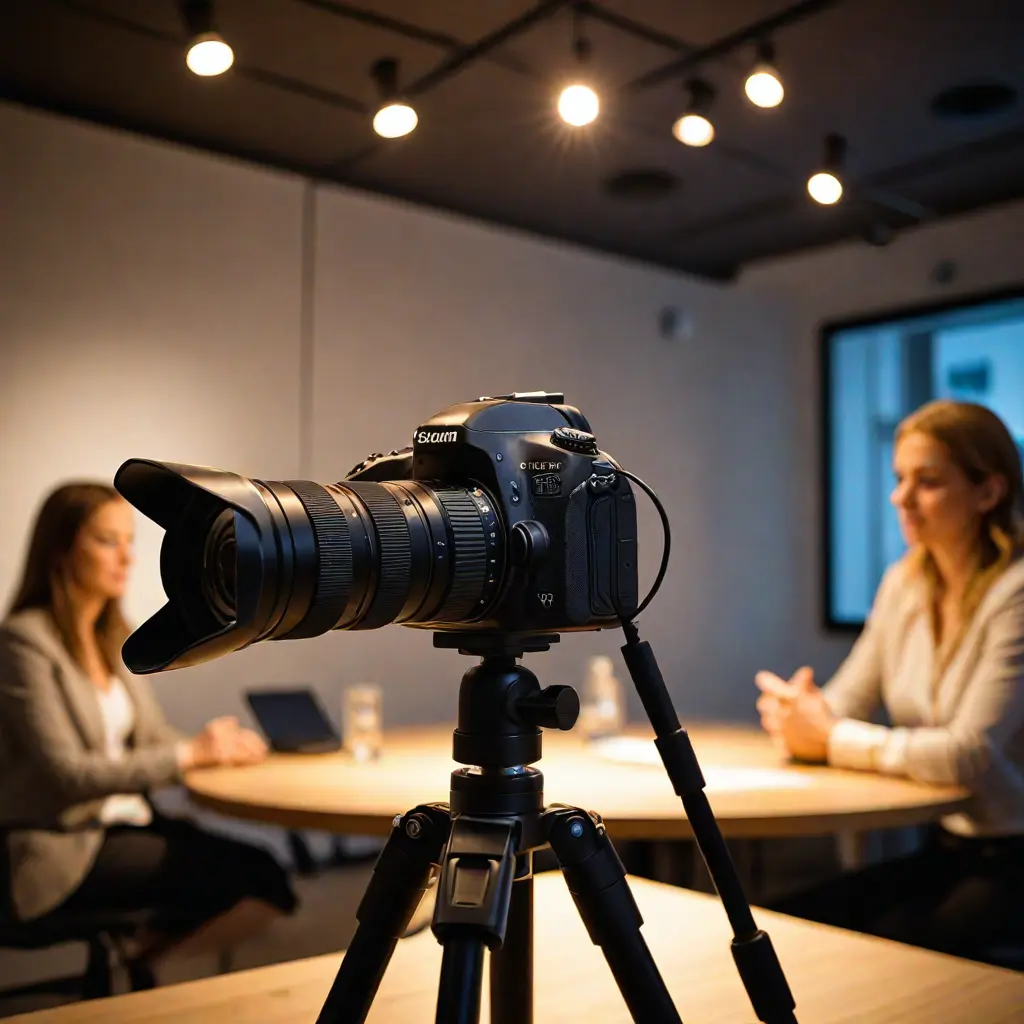
In today’s digital world, media summarization plays a crucial role in helping users consume content efficiently. Whether it’s a long podcast, an educational video, or a business meeting, summarization tools allow people to extract key insights without spending hours on playback.
But video and audio summarization are not the same—each format presents unique challenges and requires different techniques. In this blog, we’ll explore how video and audio summarization work, the key differences between them, and the challenges that come with summarizing each format.
1. What is Media Summarization?
Media summarization is the process of condensing lengthy audio or video content into a shorter, digestible version. This can be done using:
📌 Extractive Summarization – Selecting the most important segments from the content.
📌 Abstractive Summarization – Generating a human-like summary using AI language models.
Both techniques are used in audio and video summarization, but the process differs due to the nature of each media format.
2. Audio Summarization: Techniques and Challenges
Audio summarization involves extracting key information from spoken content, such as podcasts, lectures, interviews, or meetings.
🔹 Techniques Used in Audio Summarization
✅ Speech-to-Text Transcription – AI tools like Whisper (used by Dictationer) convert audio into text before summarization.
✅ Text Summarization Algorithms – Once transcribed, AI applies NLP (Natural Language Processing) to extract key sentences.
✅ Keyword Extraction – Identifies important topics, speaker mentions, and key phrases.
✅ Speaker Diarization – Recognizes and separates multiple speakers to improve summary accuracy.
🔹 Challenges in Audio Summarization
❌ Background Noise & Poor Audio Quality – AI struggles with noisy environments or low-quality recordings.
❌ Multiple Speakers & Overlapping Speech – Difficult to attribute correct information when people talk simultaneously.
❌ Speech Complexity – Understanding accents, slang, and emotions remains a challenge for AI models.
❌ Lack of Visual Context – AI must rely only on spoken words, making interpretation harder compared to video summarization.
🔹 Best Use Cases for Audio Summarization:
✔️ Podcasts & Interviews – Summarize long discussions into key insights.
✔️ Business Meetings – Convert meeting recordings into quick action points.
✔️ Lecture Notes – Help students extract key learnings from recorded classes.
3. Video Summarization: Techniques and Challenges
Video summarization is more complex than audio summarization because it involves both spoken words and visual content. AI must analyze not just speech but also on-screen actions, visuals, and contextual cues.
🔹 Techniques Used in Video Summarization
✅ Speech-to-Text Transcription & NLP – Like audio, video summarization starts with transcribing spoken words.
✅ Scene Detection & Key Frame Extraction – AI analyzes visuals to detect important scenes.
✅ Action Recognition – AI identifies important movements, gestures, and interactions.
✅ Object & Face Recognition – AI recognizes important people, text on the screen, and objects to improve relevance.
✅ Audio-Visual Fusion – AI combines both visual and audio data to generate a complete summary.
🔹 Challenges in Video Summarization
❌ High Processing Power Required – Analyzing both audio and visuals is computationally intensive.
❌ Relevance Filtering – AI struggles to determine which frames or segments are important without human guidance.
❌ Complex Scene Context – Some non-verbal cues (like facial expressions) can be difficult for AI to interpret accurately.
❌ Different Video Types Require Different Models – Summarizing a news broadcast vs. a sports event requires different techniques.
🔹 Best Use Cases for Video Summarization:
✔️ YouTube & TikTok Summaries – Condense long videos into quick highlights.
✔️ Webinars & Online Courses – Extract key moments for fast learning.
✔️ Security Footage Analysis – Identify important events from long surveillance videos.
4. Key Differences: Audio vs. Video Summarization
FeatureAudio SummarizationVideo Summarization
Input Data
Speech Only
Speech + Visual Content
Processing Complexity
Lower
Higher (Requires scene analysis)
Key Techniques
Speech-to-Text, NLP
Speech-to-Text, Object Detection, Scene Segmentation
Challenges
Noise, speaker overlap
High computational cost, irrelevant frames
Output
Text summary
Text + Video highlights
5. The Future of Media Summarization
🚀 Multimodal AI Models – Future AI models will be able to analyze speech, video, and text simultaneously, improving summary quality.
🚀 Real-Time Summarization – AI-powered tools like Dictationer will allow instant summarization of live meetings, lectures, and videos.
🚀 Personalized Summarization – Users will be able to customize summaries based on preferences (e.g., "focus on business insights" or "extract emotional tone").
As AI advances, summarization will become more accurate, efficient, and personalized, helping users save time and stay informed in an era of information overload.
Final Thoughts
Both audio and video summarization are essential tools for efficient content consumption, but each presents unique challenges and requires different AI techniques.
✅ Use audio summarization for podcasts, meetings, and voice-based content.
✅ Use video summarization for YouTube, webinars, and visually rich content.
🚀 Want to experience AI-powered media summarization? Try Dictationer today for accurate speech-to-text, transcription, and AI-generated summaries!
Share and Earn Credits!
Share this link and earn credits when others visit or register.
Share anywhere you like - SNS, messaging apps, or any platform of your choice!
Learn more about Free Credit